Integrated Molecular and Separation System Design for Hydrofluorocarbon Recycling
Enabling Hydrofluorocarbon (HFC) Recycling with Innovative Separations
Mandated by the 1987 Montreal Protocol, chlorofluorocarbon (CFC) refrigerants have been gradually replaced by HFCs to prevent ozone depletion. Many of these second-generation HFC mixtures, however, have a high global warming potential (GWP) and the 2016 Kigali agreement ordered their gradual phase-out. Due to the often azeotropic compositions of these HFC refrigerants, existing separation methods for removing the high GWP components are currently infeasible or impractical, but it is wasteful to incinerate HFC mixtures as some HFC components have low GWPs and can be recycled.
We hypothesize that custom Ionic Liquids (ILs) can be designed to remove low GWP HFC components from specific HFC mixtures. Traditionally, Computer-Aided Molecular Design (CAMD) methods would be used to screen the millions of potential ILs for properties specific to a particular HFC separation. However, these methods often fail for complex IL molecules and lead to uncertainty as to which candidate IL design should be promoted for experimental analysis.
To overcome this issue, we propose augmenting traditional CAMD methods with molecular simulations for more reliable IL screening. We develop a data-to-process design workflow which can rapidly develop and optimize an HFC mixture separation process using a specific IL, with a feedback loop which enables guidance for studying optimal ILs. Additionally, we use data science tools to dive into the molecular, thermodynamic, process, and techno-economic models which are used in our data-to-design workflow, providing a more robust understanding of multi-scale design decisions made in the workflow.
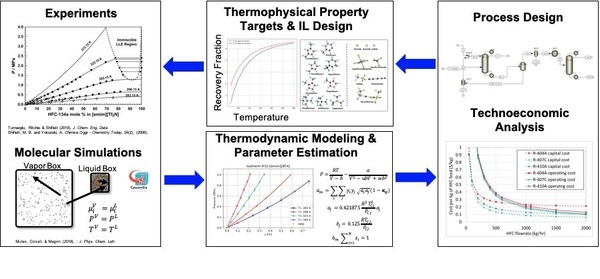
Harnessing Molecular Simulation as an Accurate Data Generation Technique
Molecular simulations, which use classical molecular models, called force fields, to calculate the physical properties of molecules, can be used as a guide for focusing on specific IL designs within CAMD and as a method of quickly generating data for our data-to-design workflow. However, harnessing molecular simulation in a predictive capacity for screening molecular design space requires accurate force fields. Developing and optimizing force fields is challenging, often limited by the computational cost of simulations and the calculation of the objective function, or the error between the simulation result and the property of interest. We propose a machine learning (ML)-enabled automated force field optimization framework which reduces the reliance on expensive simulations for model calibration. Specifically, we show integrating Gaussian Process (GP) surrogate models with Baysian optimization facilitates rapid tuning of force fields and provides a quick and efficient route to highly accurate, physics-based molecular models. As further capabilities are developed, this tool will be harnessed to rapidly calibrate molecular models for HFCs, ILs, and systems of interest and multiscale design schemes to facilitate rapid molecular and process design and optimization.
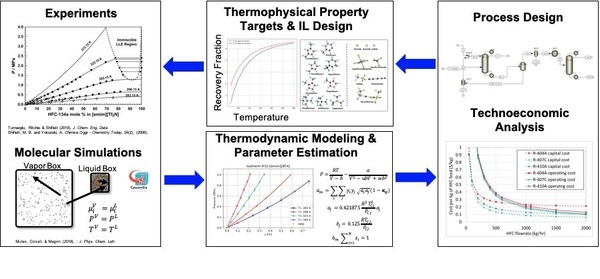
Related Publications
Bridgette J. Befort, Ryan S. DeFever, Garrett M. Tow, Alexander W. Dowling, Edward J. Maginn (2021). Machine Learning Directed Optimization of Classical Molecular Modeling Force Fields, J. Chem. Inf. Model, 61(9), p. 440-4414. [preprint] [link]
Alejandro Garciadiego, Bridgette J. Befort, Gabriela Franco, Mozammel Mazumder, and Alexander W. Dowling (2022). What Data Are Most Valuable to Screen Ionic Liquid Entrainers for Hydrofluorocarbon Refrigerant Reuse and Recycling? (under review)
Alejandro Garciadiego, Mozammel Mazumder, Bridgette J. Befort and Alexander W. Dowling. Techno-economic Analysis of Extractive Distillation of Ternary Hydrofluorocarbon Mixtures using Ionic Liquid Entertainers (2022). 14th International Symposium on Process Systems Engineering (PSE2021+). Ed. by Y. Yamashita, M. Kano. [file]
Bridgette J. Befort, Ryan S. DeFever, Edward J. Maginn, Alexander W. Dowling. Machine Learning-Enabled Optimization of Force Fields for Hydrofluorocarbons (2022). 14th International Symposium on Process Systems Engineering (PSE2021+). Ed. by Y. Yamashita, M. Kano. [file]
Kalin R. Baca, Greta M. Olsen, Lucia Matamoros Valenciano, Madelyn G. Bennett, Dorothy M. Haggard, Bridgette J. Befort, Alejandro Garciadiego, Alexander W. Dowling, Edward J. Maginn, Mark B. Shiflett (2022), Phase Equilibria and Diffusivities of HFC-32 and HFC-125 in Ionic Liquids for the Separation of R-410A. ACS Suistainable Chemistry & Engineering, 10(2), p. 816-830. [link]
Ana Rita C. Morais, Abby Harders, Kalin Baca, Greta M. Olson, Mark B. Shiflett, Bridgett Befort, Alexander W. Dowling, Edward J. Maginn (2020). Phase Equilibria, Diffusivities, and Equation of State Modeling of HFC-32 and HFC-125 in Imidazolium-based Ionic Liquids for the Separation of R-410A, Industrial Engineering & Chemistry Research, 59(40), p. 18222–18235. [link]
Support from Notebaert Fellowship (B. Befort), MERITS Fellowship (M. Carlozo), the University of Notre Dame, and NSF Grant No. 1917474.